PROJECTS
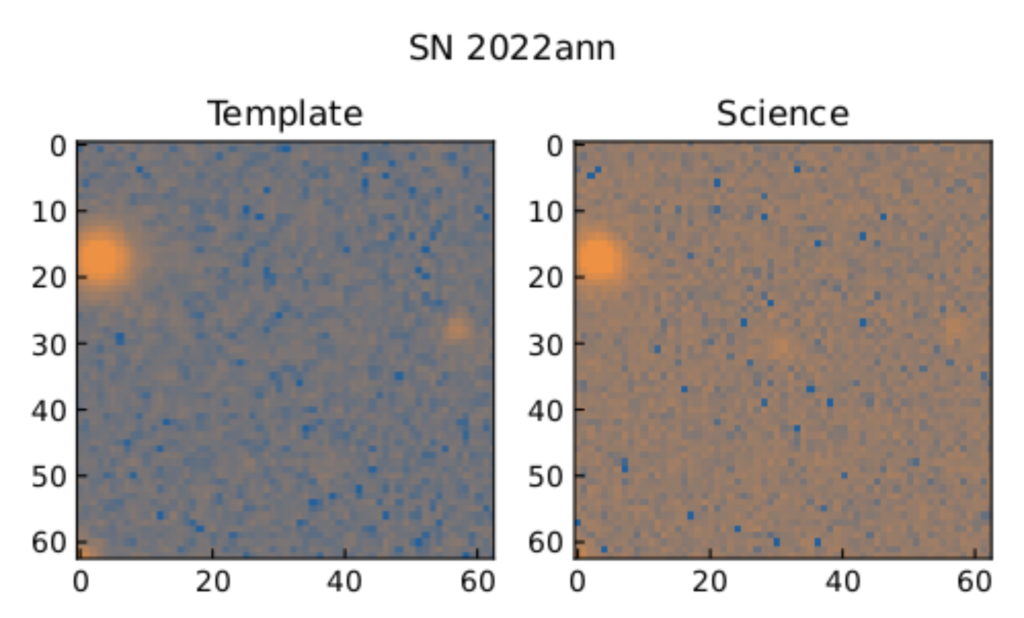
25 - ELEPHANT: ExtragaLactic alErt Pipeline for Hostless AstroNomical Transients
ELEPHANT represents an effective strategy to filter extragalactic events within large and complex astronomical alert streams. There are many applications for which this pipeline will be useful, ranging from transient selection for follow-up to studies of transient environments. We find that less than 2% of all analyzed transients are potentially hostless. Among them, approximately 10% have a spectroscopic class reported on TNS, with Type Ia supernova being the most common class, followed by SLSN. Among the hostless candidates retrieved by our pipeline, there was SN 2018ibb, which has been proposed to be a PISN candidate; and SN 2022ann, one of only five known SNe Icn. When no class is reported on TNS, the dominant classes are QSO and SN candidates, the former obtained from SIMBAD and the latter inferred using the Fink ML classifier.
24 - Are classification metrics good proxies for SN Ia cosmological constraining power?
We emulate photometric SN Ia cosmology samples with controlled contamination rates of individual contaminant classes and evaluate each of them under a set of classification metrics. We then derive cosmological parameter constraints from all samples under two common analysis approaches and quantify the impact of contamination by each contaminant class on the resulting cosmological parameter estimates. We observe that cosmology metrics are sensitive to both the contamination rate and the class of the contaminating population, whereas the classification metrics are insensitive to the latter. We therefore discourage exclusive reliance on classification-based metrics for cosmological analysis design decisions, e.g. classifier choice, and instead recommend optimizing using a metric of cosmological parameter constraining power.
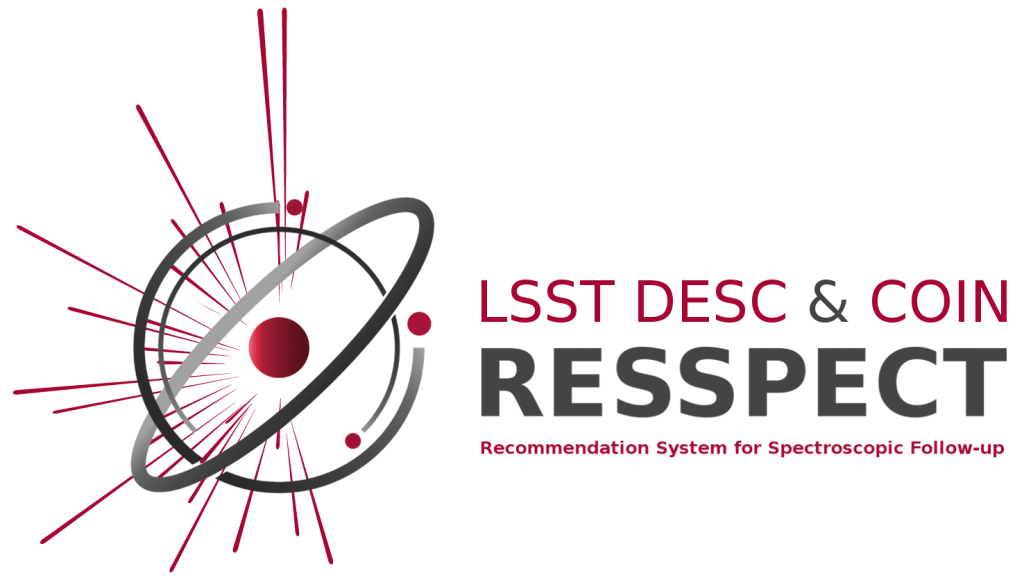
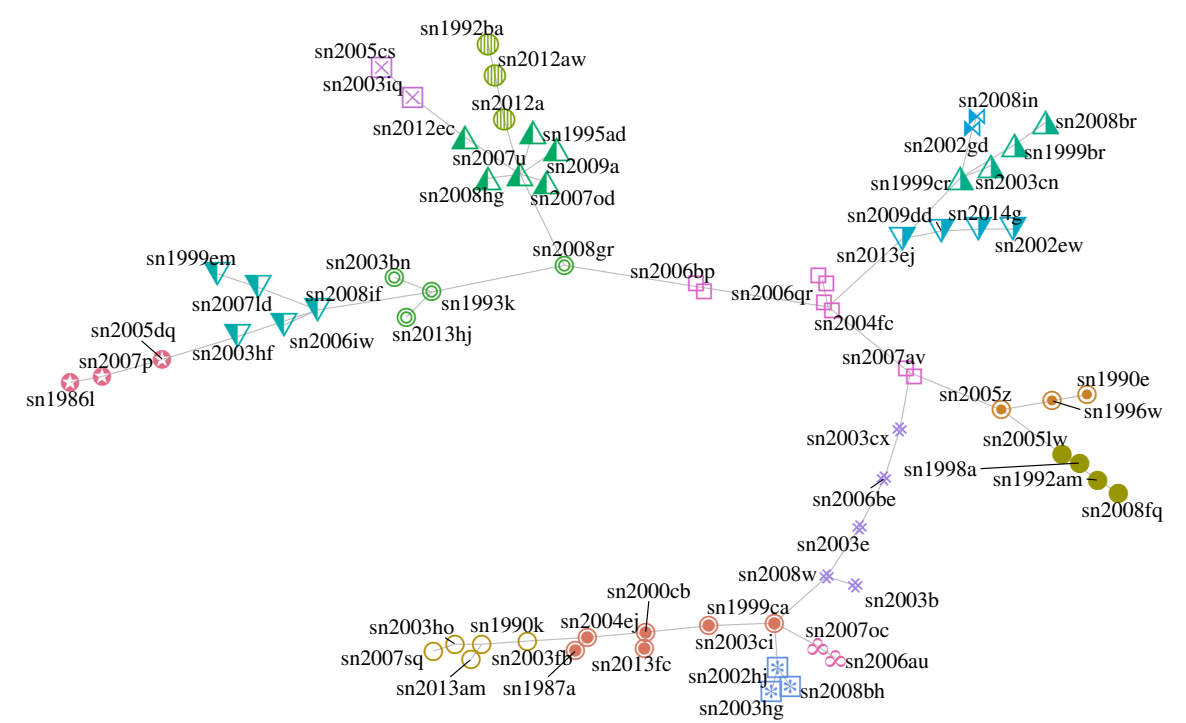
23 - A graph-based spectral classification of SN-II
This work presents new data-driven classification heuristics for spectral data based on graph theory. As a case in point, we devise a spectral classification scheme of Type II supernova (SNe II) as a function of the phase relative to the V -band maximum light and the end of the plateau phase. Our classification method naturally identifies outliers and arranges the different SNe in terms of their major spectral features. The automated classification naturally reflects the fast evolution of Type II SNe around the maximum light while showcasing their homogeneity close to the end of the plateau phase. The scheme we develop could be more widely applicable to unsupervised time series classification or characterization of other functional data.
22 - Spectroscopic Confirmation of a population of Isolated, Intermediate-Mass YSOs
The Spitzer/IRAC Candidate YSO (SPICY) catalog is one of the largest compilations of such objects (~120,000 candidates in the Galactic midplane). Many SPICY candidates are spatially clustered, but, perhaps surprisingly, approximately half the candidates appear spatially distributed. To better characterize this unexpected population and confirm its nature, we obtained Palomar/DBSP spectroscopy for 26 of the optically-bright (G<15 mag) "isolated" YSO candidates. We confirm the YSO classifications of all 26 sources based on their positions on the Hertzsprung-Russell diagram, H and Ca II line-emission from over half the sample, and robust detection of infrared excesses. This implies a contamination rate of <10% for SPICY stars that meet our optical selection criteria.
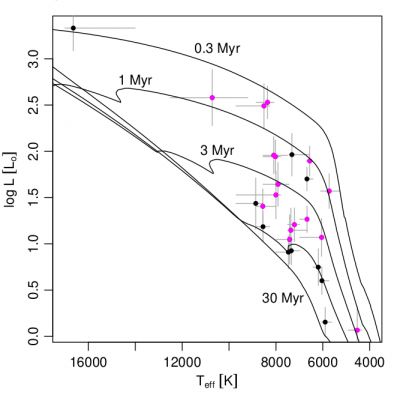
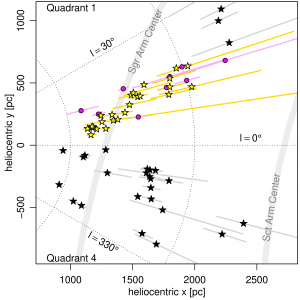
21 - A high pitch angle structure in the Sagittarius Arm
We map the 3D locations and velocities of star-forming regions in a segment of the Sagittarius Arm using young stellar objects (YSOs) from the Spitzer/IRAC Candidate YSO (SPICY) catalog to compare their distribution to models of the arm. Distances and velocities for these objects are derived from Gaia EDR3 astrometry and molecular line surveys. We infer parallaxes and proper motions for spatially clustered groups of YSOs and estimate their radial velocities from the velocities of spatially associated molecular clouds. The observed 56◦ pitch angle is remarkably high for a segment of the Sagittarius Arm. We discuss possible interpretations of this feature as a substructure within the lower pitch angle Sagittarius Arm, as a spur, or as an isolated structure.
20 - Dawn of Stars
Dawn of Stars tells the story of how stars are formed. Most stars are born in groups which are truly stellar nurseries composed by clouds of dust and gas. This birth process is full of episodes, some of which are represented musically in the four parts of this piece. This was the first art-related project to be developed by COIN, in March 2021, in collaboration with Rodrigo Roriz Teodoro, as part of his graduation project to obtain the degree of Master in music composition awarded by the Marshall University (USA).
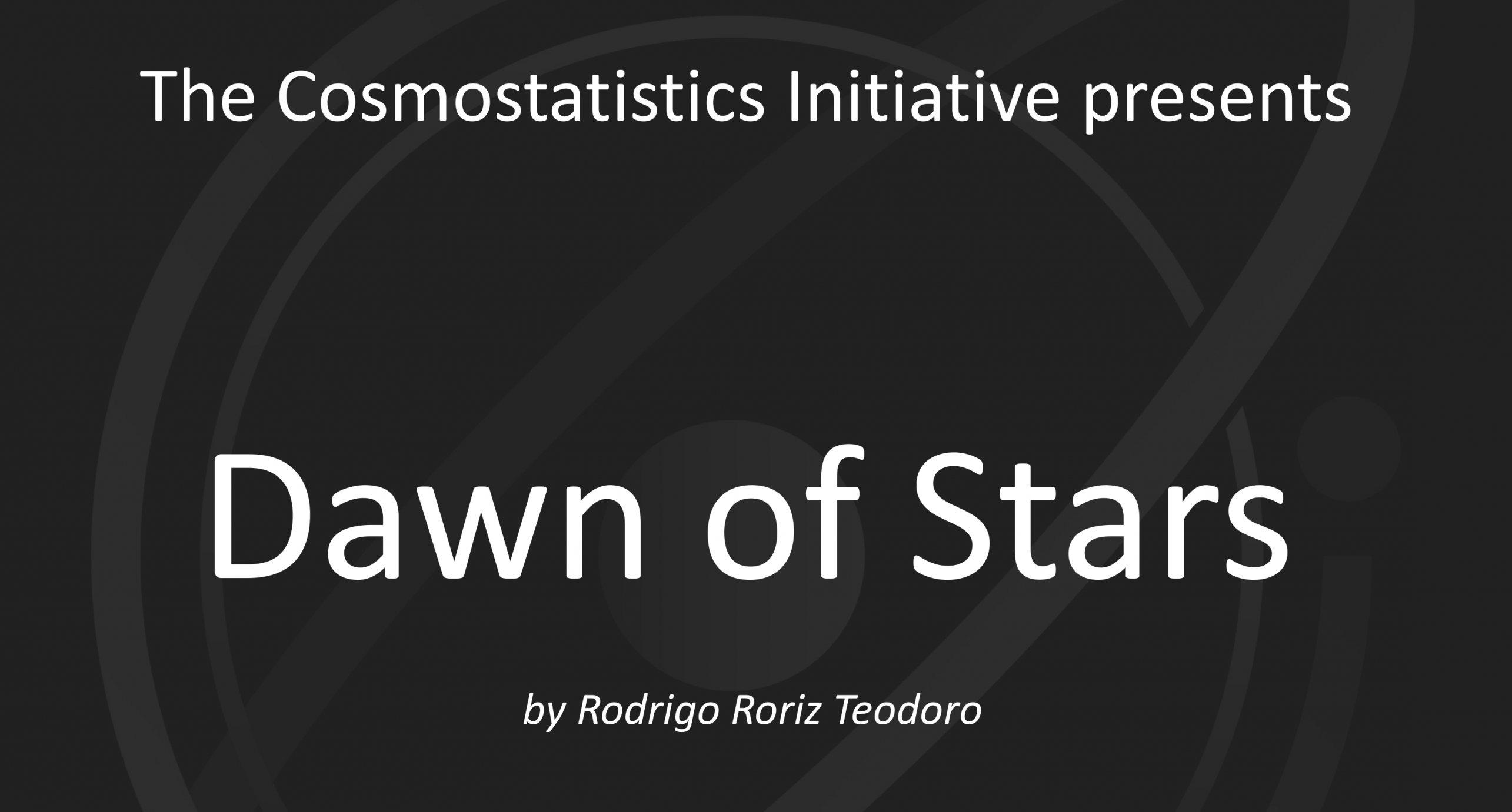
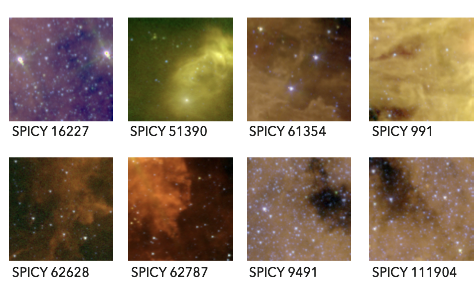
19 - SPICY: The Spitzer/IRAC Candidate YSO Catalog
We present ~120,000 candidate young stellar objects (YSOs) based on surveys of the Galactic midplane between l ∼ 255° and 110°, including the GLIMPSE I, II, and 3D, Vela-Carina, Cygnus X, and SMOG surveys (613 square degrees), augmented by near-infrared catalogs. We employed a classification scheme that uses the flexibility of a tailored statistical learning method and curated YSO datasets to take full advantage of IRAC’s spatial resolution and sensitivity in the mid-infrared ∼3–9 μm range. We also identify areas of IRAC color space associated with objects with strong silicate absorption or polycyclic aromatic hydrocarbon emission. Spatial distributions and variability properties help corroborate the youthful nature of our sample..
18 - Active Learning with RESSPECT
The Recommendation System for Spectroscopic follow-up (RESSPECT) project aims to enable the construction of optimized training samples for the Rubin Observatory Legacy Survey of Space and Time (LSST), taking into account a realistic description of the astronomical data environment. In this work, we test the robustness of active learning techniques in a realistic simulated astronomical data scenario. Our experiment takes into account the evolution of training and pool samples, different costs per object, and two different sources of budget. Results show that traditional active learning strategies significantly outperform random sampling.
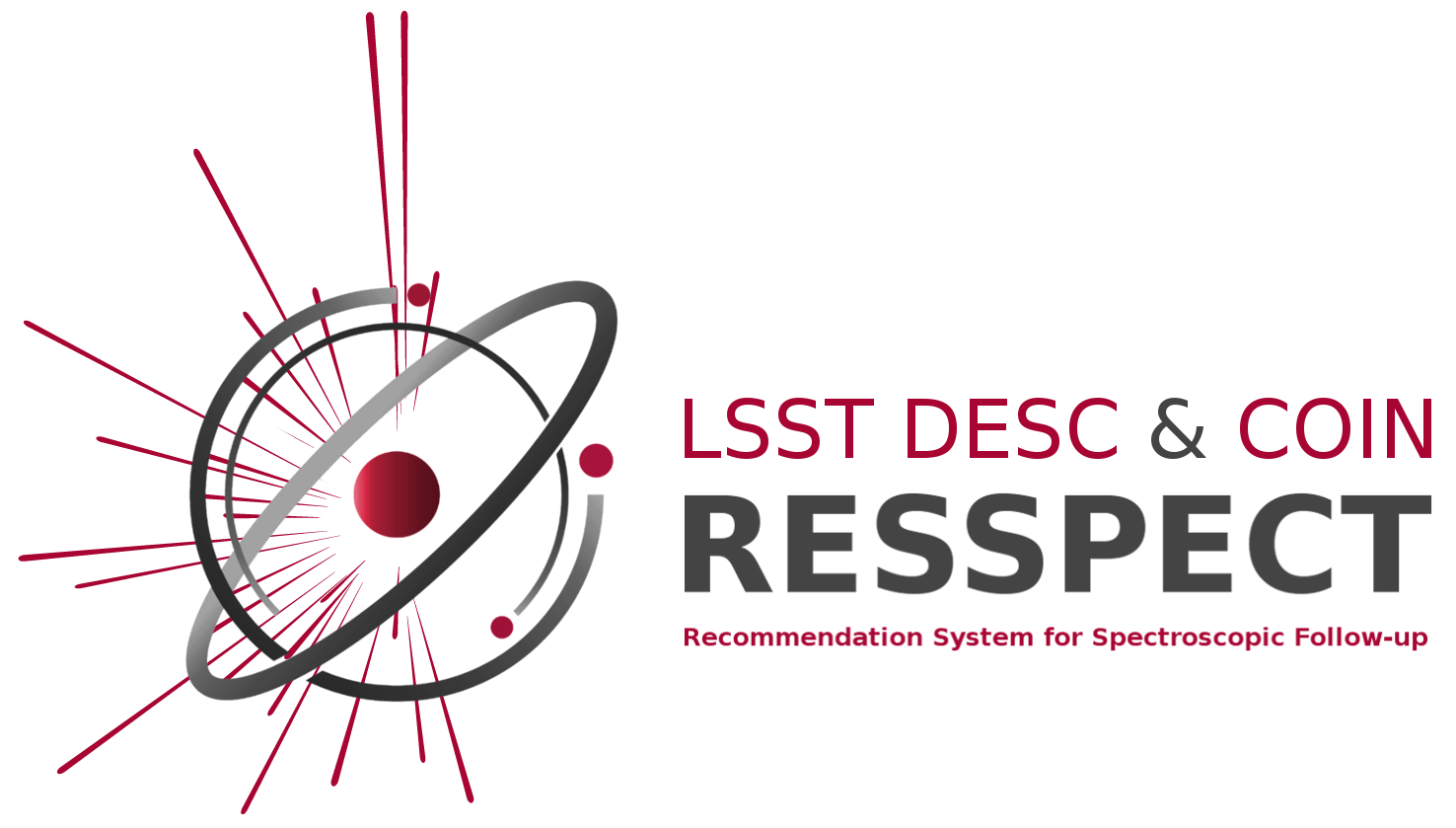
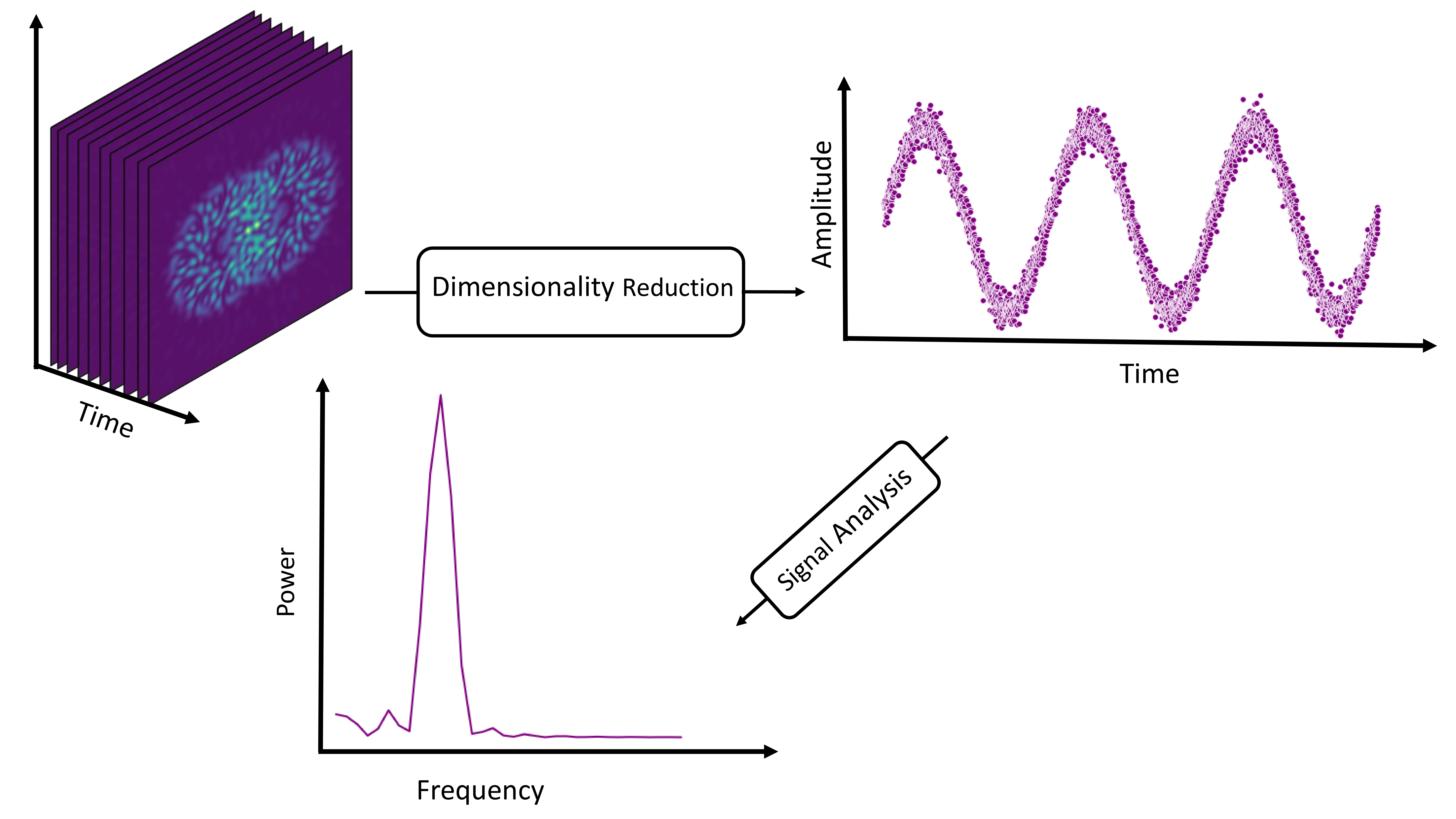
17 - Periodic Astrometric Signal Recovery through Convolutional Autoencoders
Astrometric detection involves a precise measurement of stellar positions, and is widely regarded as the leading concept presently ready to find earth-mass planets in temperate orbits around nearby sun-like stars. The TOLIMAN space telescope is a low-cost, agile mission concept dedicated to narrow-angle astrometric monitoring of bright binary stars.In this paper we demonstrate that a Deep Convolutional Auto-Encoder is able to detected signals from simplified simulations of the TOLIMAN data and we present the full experimental pipeline to recreate out experiments from the simulations to the signal analysis.
16 - Ridges in the Dark Energy Survey
Cosmic voids play an important role in our attempt to model the large-scale structure of the Universe. In this paper, we apply it to 2D weak-lensing mass density maps to identify curvilinear filamentary structures. Our results demonstrate the viability of ridge estimation as a precursor for denoising weak lensing quantities to recover the large-scale structure, paving the way for a more versatile and effective search for troughs.
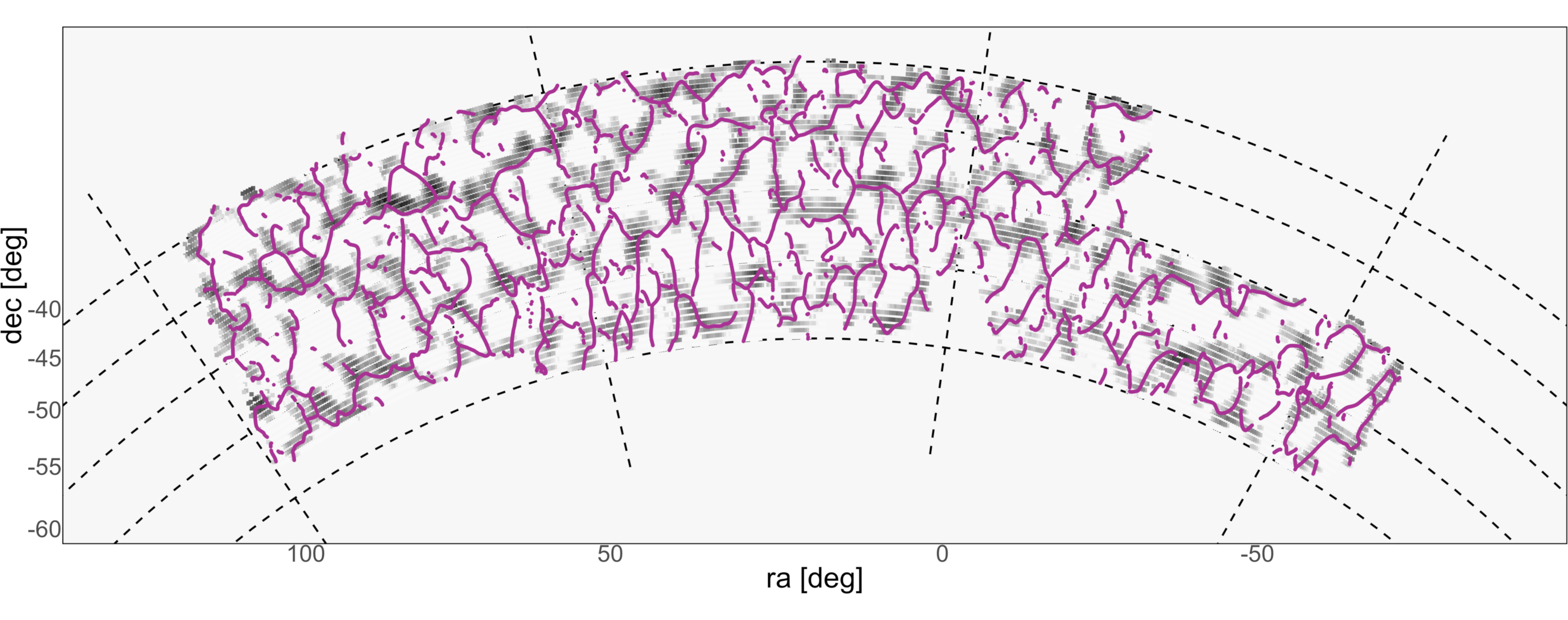
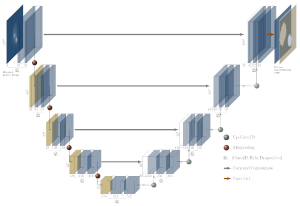
15 - Photometry of high-redshift blended galaxies using deep learning
This work explores the use of deep neural networks to estimate the photometry of blended pairs of galaxies in monochrome space images, similar to the ones that will be delivered by the Euclid space telescope. Using a clean sample of isolated galaxies from the CANDELS survey, we artificially blend them and train two different network models to recover the photometry of the two galaxies. We show that our approach can recover the original photometry of the galaxies before being blended with ~7% accuracy without any human intervention and without any assumption on the galaxy shape.
14 - Dark energy equation of state imprint on supernova data
This work determines the degree to which a standard Lambda-CDM analysis based on type Ia supernovae can identify deviations from a cosmological constant in the form of a redshift-dependent dark energy equation of state w(z). We demonstrate that a standard type Ia supernova cosmology analysis has limited sensitivity to extensive redshift dependencies of the dark energy equation of state. In addition, we report that larger redshift-dependent departures from a cosmological constant do not necessarily manifest easier-detectable incompatibilities with the Lambda-CDM model.
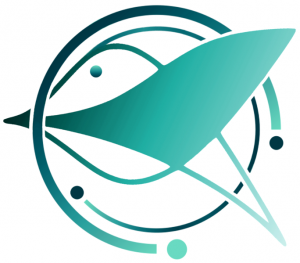

13 - Hurdle and Generalized Additive Models
We show that the baryonic fraction and the rate of ionizing photons appear to have a larger impact on fesc than previously thought. A naive univariate analysis of the same problem would suggest smaller effects for these properties and a much larger impact for the specific star formation rate, which is lessened after accounting for other galaxy properties and non-linearities in the statistical model.
12 - Incompleteness of nearby cluster population
We report the discovery of 41 new stellar clusters. This represents an increment of at least 20% of the previously known OC population in this volume of the Milky Way. We also report on the clear identification of NGC 886, an object previously considered an asterism. This letter challenges the previous claim of a near-complete sample of open clusters up to 1.8 kpc. Our results reveal that this claim requires revision, and a complete census of nearby open clusters is yet to be found.
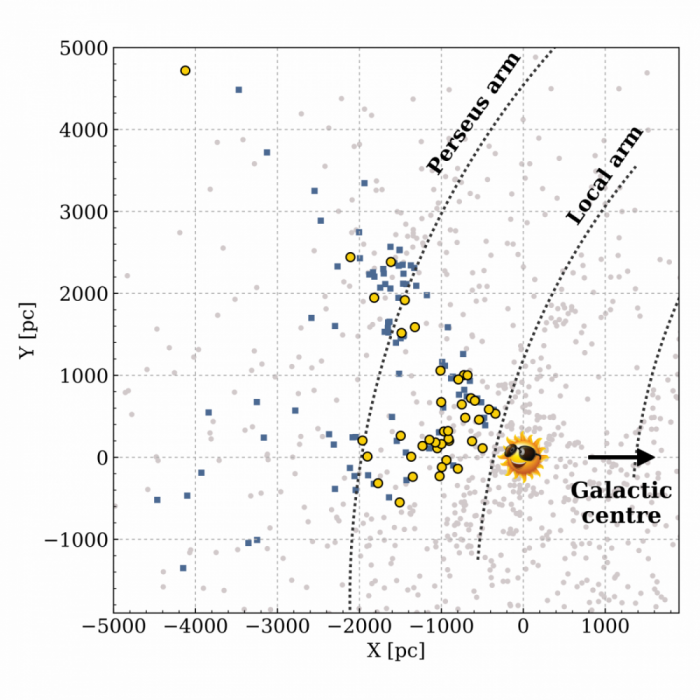
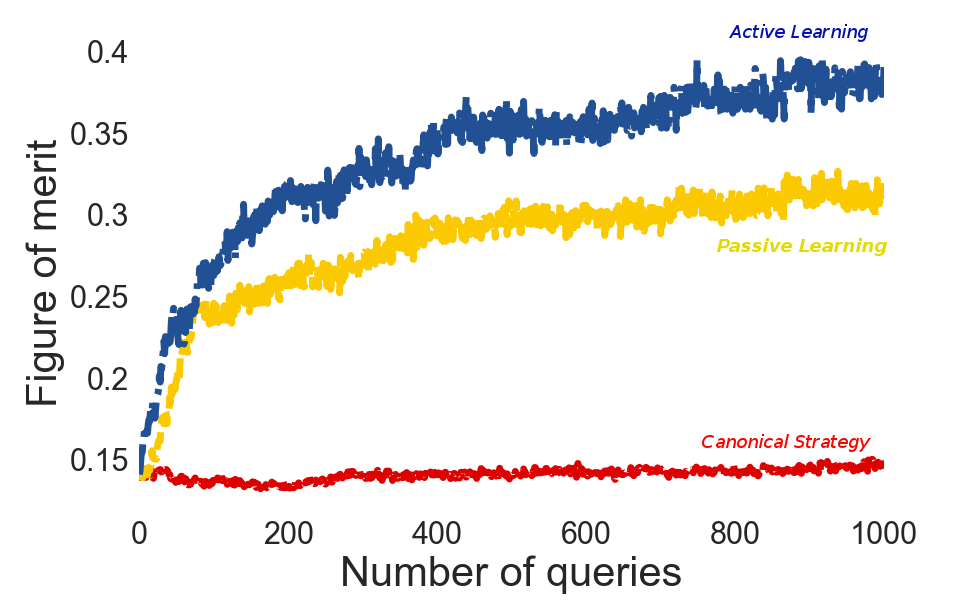
11 - Active Learning for Supernova Photometric Classification
Active Learning is a class of algorithms that aims to minimize labeling costs by identifying a few, carefully chosen, objects which have high potential in improving a given machine learning classifier. In this project, we show how Active Learning can be used as a tool for optimizing the construction of spectroscopic samples for supernova photometric classification.
10 - Integrated Nested Laplace Approximation (INLA)
We introduce a novel technique to model IFS datasets, which treats the observed galaxy properties as manifestations of an unobserved Gaussian Markov random field. The method is computationally efficient, resilient to the presence of low-signal-to-noise regions, and uses an alternative to Markov Chain Monte Carlo for fast Bayesian inference - the Integrated Nested Laplace Approximation. The proposed Bayesian approach enables the creation of synthetic images, recovery of areas with bad pixels, and an increased power to detect structures in datasets subject to substantial noise and/or sparsity of sampling.
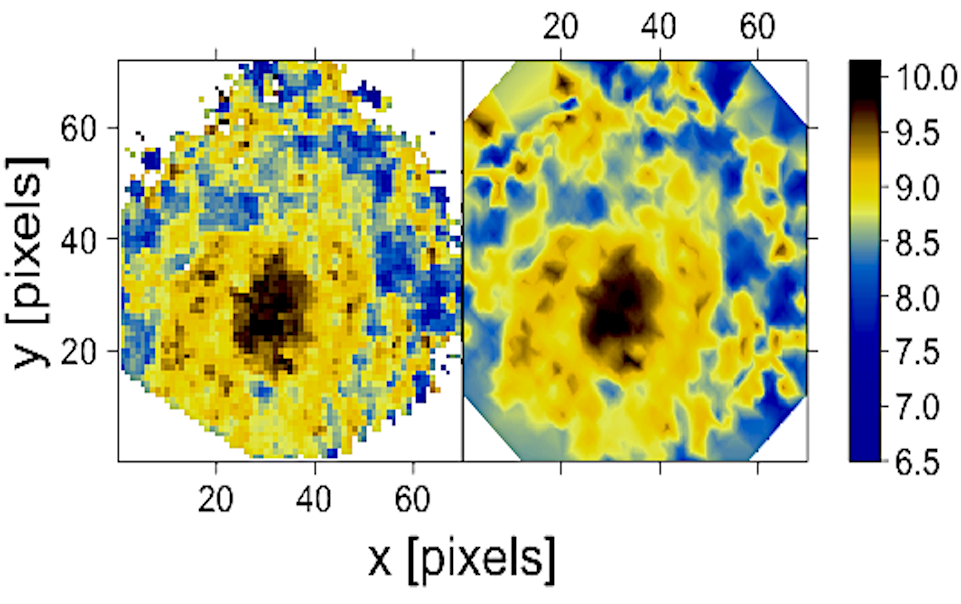
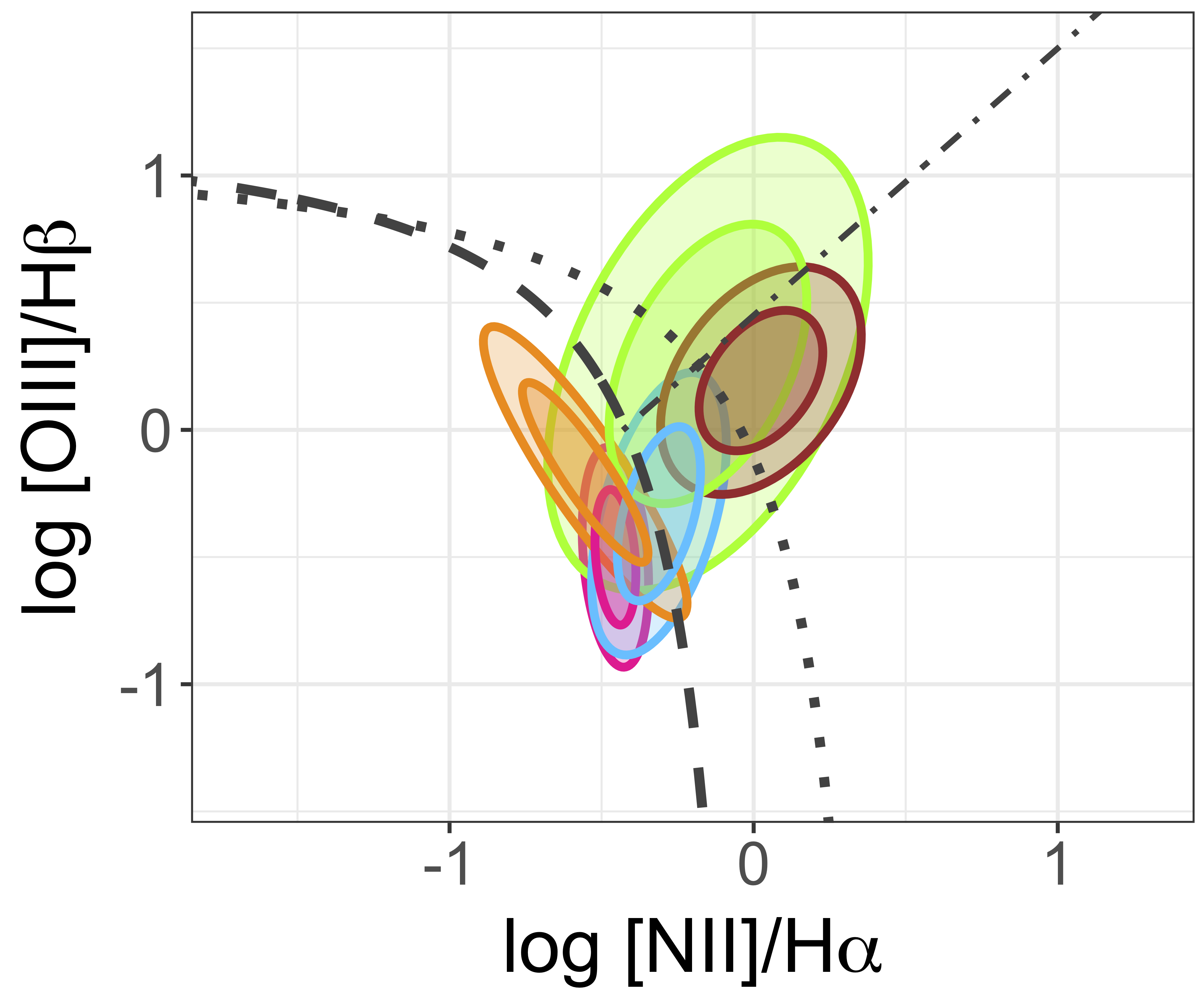
9 - Gaussian Mixture Models
Here, we show how to use a Gaussian mixture model (GMM) to jointly analyse two traditional emission-line classification schemes of galaxy ionization sources: the Baldwin-Phillips-Terlevich (BPT) and the WHAN diagrams, using spectroscopic data from the Sloan Digital Sky Survey Data Release 7 and SEAGal/STARLIGHT datasets. We apply a GMM to empirically define classes of galaxies in a three-dimensional space spanned by the log [OIII]/H-beta, log [NII]/H-alpha, and log EW(H-alpha) optical parameters. We demonstrate the potential of our methodology to recover/unravel different objects inside the wilderness of astronomical datasets, without lacking the ability to convey physically interpretable results; hence being a precious tool for maximum exploitation of the ever-increasing astronomical surveys.
8 - Representativeness in Machine Learning applications for photometric redshifts
We present two galaxy catalogues built to enable a more demanding and realistic test of photo-z methods. We demonstrate the potential of these catalogues by submitting them to the scrutiny of different photo-z methods, including machine learning (ML) and template fitting approaches. Our catalogues represent the first controlled environment allowing a straightforward implementation of such tests.
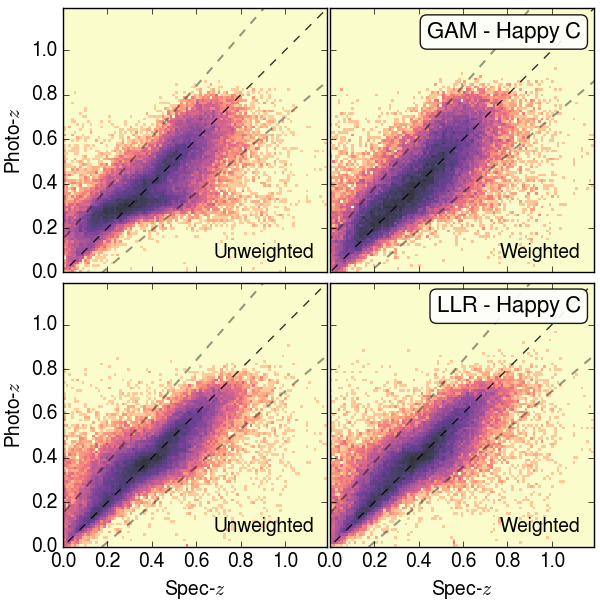
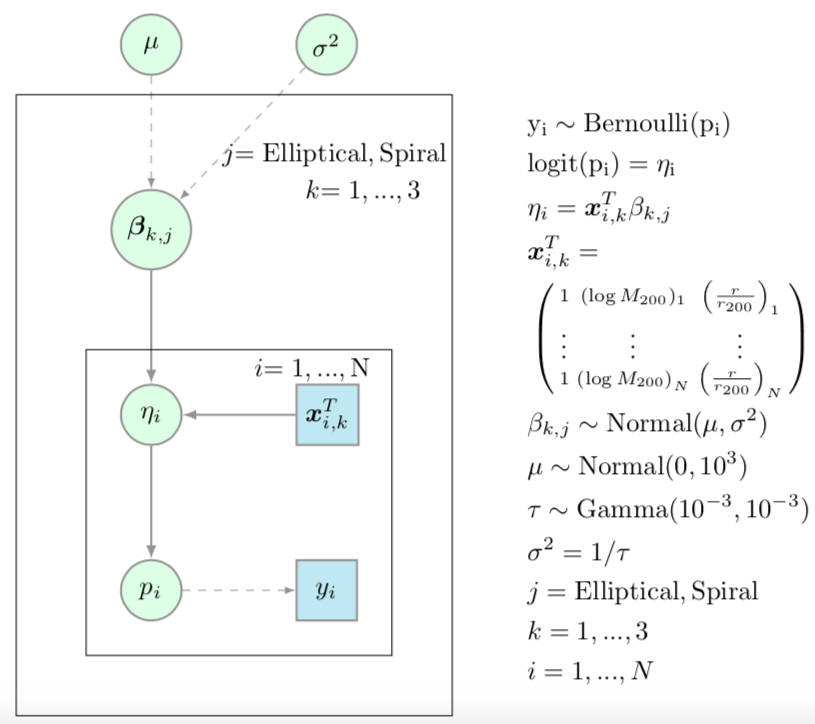
7 - Hierarchical Bayesian Models
We developed a hierarchical Bayesian model to investigate how the presence of Seyfert activity relates to their environment. In elliptical galaxies, our analysis indicates a strong correlation of Seyfert-AGN activity with the cluster centric distance, and a weaker correlation with the mass of the host. In spiral galaxies these trends do not appear, suggesting that the link between Seyfert activity and the properties of spiral galaxies are independent of the environment.
6 - Dimensionality Reduction And Clustering for Unsupervised Learning in Astronomy (DRACULA)
DRACULA classifies objects using dimensionality reduction and clustering. The code has an easy interface and can be applied to separate several types of objects. It is based on tools developed in scikit-learn, with Deep Learning usage requiring also the H2O package. We show how it can be used to identify sub-classes of type Ia supernovae.
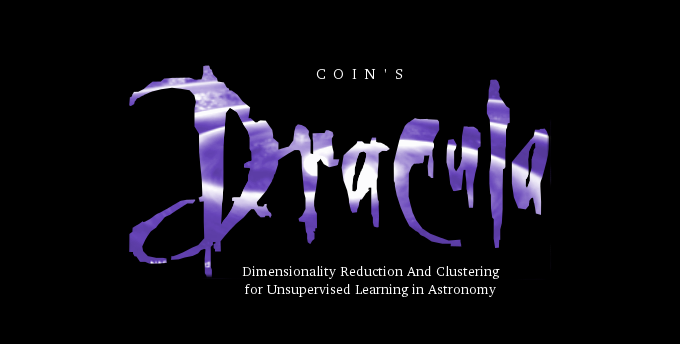
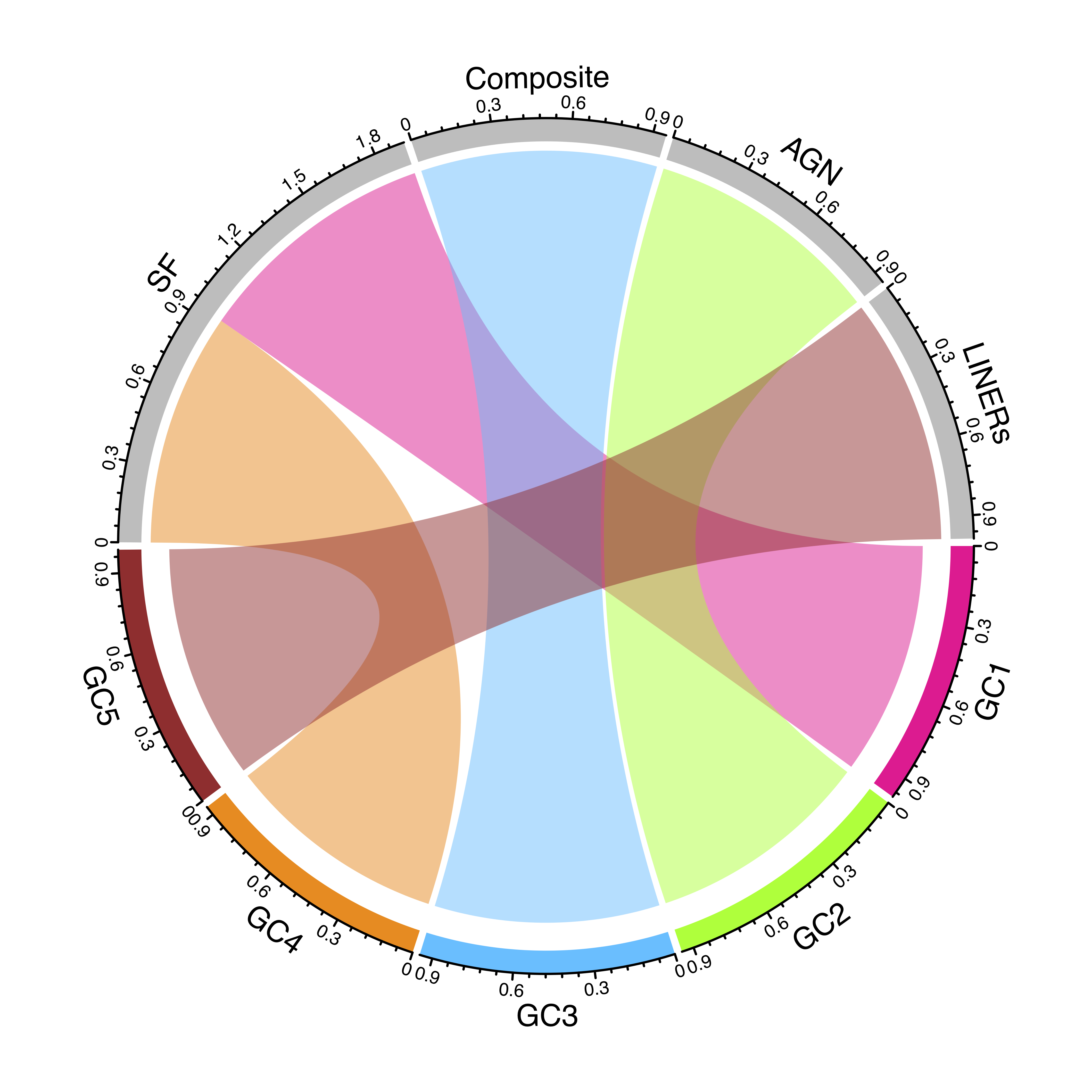
5 - Analysis of Multi-dimensional Astronomical DAta sets (AMADA)
AMADA allows an iterative exploration and information retrieval of high-dimensional data sets. This is done by performing a hierarchical clustering analysis for different choices of correlation matrices and by doing a principal components analysis in the original data. Additionally, AMADA provides a set of modern visualisation data-mining diagnostics. The user can switch between them using the different tabs.
4 - Approximate Bayesian Computation (CosmoABC)
Approximate Bayesian Computation (ABC) enables parameter inference for complex physical systems in cases where the true likelihood function is unknown, unavailable, or computationally too expensive. Here we present COSMOABC, a Python ABC sampler featuring a Population Monte Carlo variation of the original ABC algorithm, which uses an adaptive importance sampling scheme.
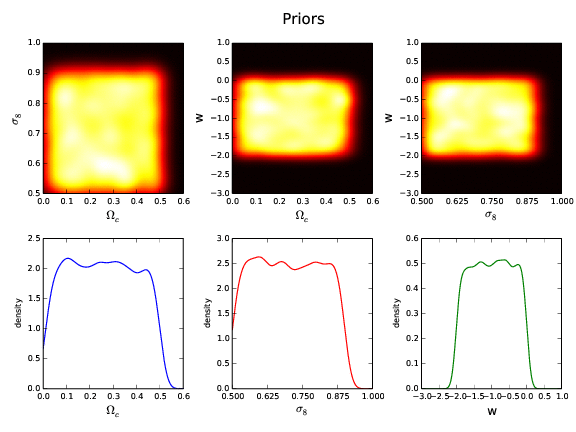
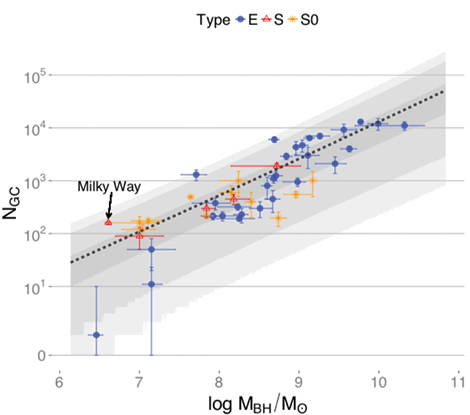
3 - GLM III: Negative Binomial Regression
We elucidate the potential of the class of GLMs which handles count data. The size of a galaxy's globular cluster (GC) population (NGC) is a prolonged puzzle in the astronomical literature. It falls in the category of count data analysis, yet it is usually modelled as if it were a continuous response variable. We have developed a Bayesian negative binomial regression model to study the connection between NGC and the host galaxy properties.
2 - GLM II: Gamma Regression
We present a gamma regression model as a fast alternative method for estimating the photometric redshifts of galaxies from their multi-wavelength photometry. Using the gamma family with a log link function we predict redshifts from the PHoto-z Accuracy Testing simulated catalogue and a subset of the Sloan Digital Sky Survey from Data Release 10.
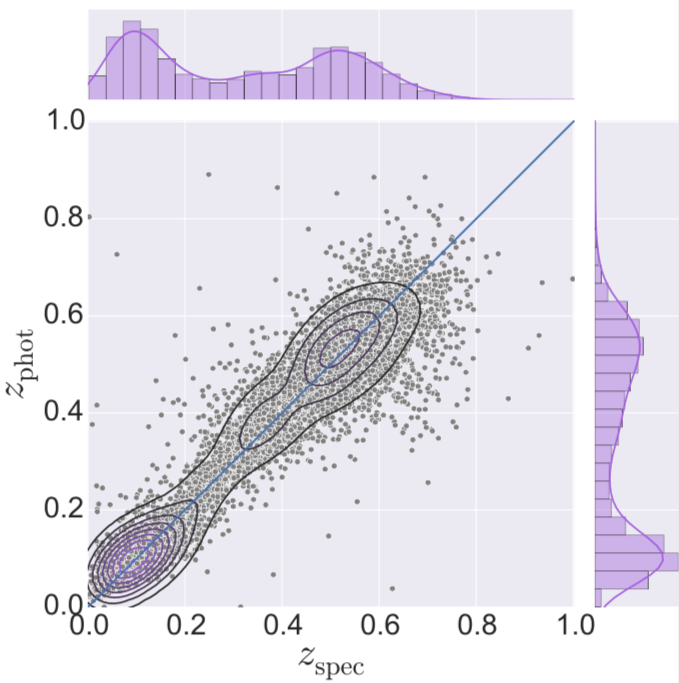
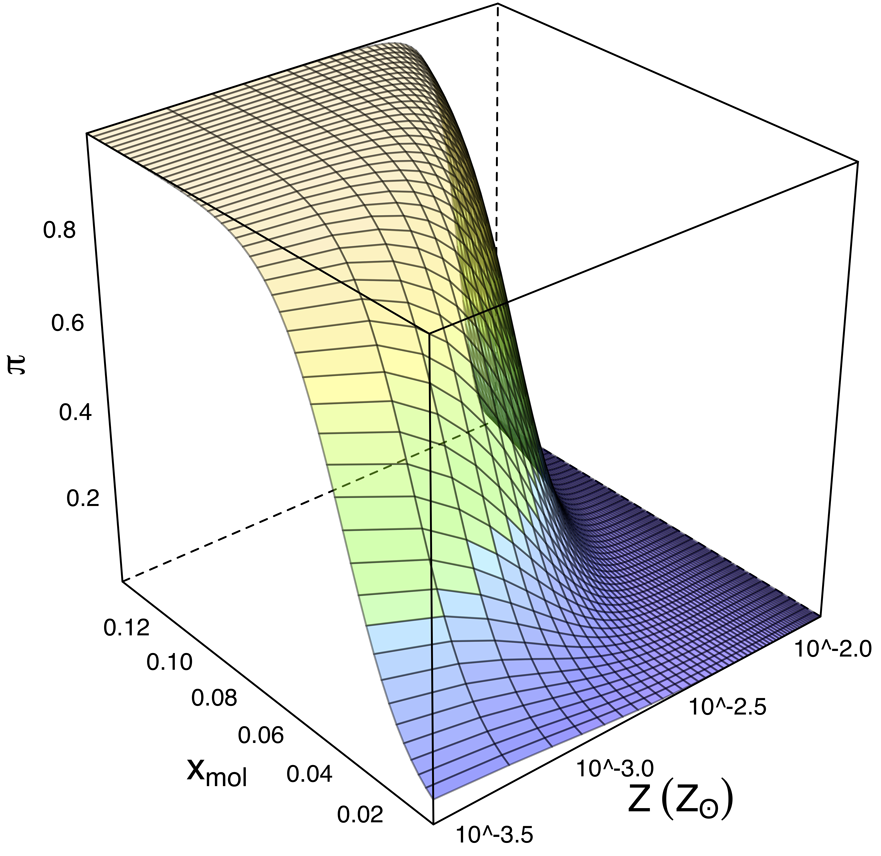
1 - GLM I: Binomial Regression
We elucidate the potential of the so-called logit and probit regression techniques, from both a maximum likelihood and a Bayesian perspective. As a case in point, we explore the conditions of star formation activity and metal enrichment in primordial minihaloes from cosmological hydro-simulations including detailed chemistry, gas physics, and stellar feedback.